How Two AI Pioneers Won the Physics Nobel Prize: A Story of Innovation and Responsibility
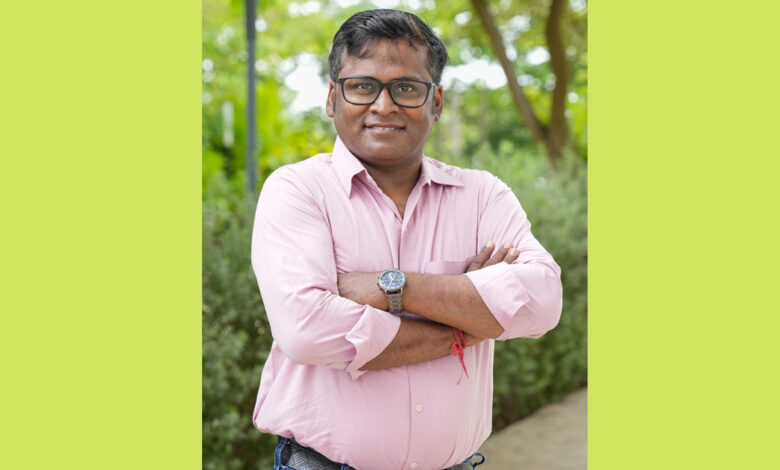
Dr. Abhijit Dasgupta, Assistant Professor, Department of Computer Science and Engineering, SRM University-AP
Algorithms are becoming a fundamental part of our lives, and the awarding of the recent Nobel Prize in Physics to John J. Hopfield and Geoffrey E. Hinton for their contributions to artificial neural networks highlights how foundational research has the power to revolutionize the future. Hinton, often called the “godfather of Artificial Intelligence (AI),” and Hopfield, a pioneer in understanding memory, have made groundbreaking contributions that have not only redefined technology but also changed how we think about intelligence. Thus, in this year, the Nobel Committee of Physics has decided to award technology driven by fundamental results of Physics rather than fundamental results only.
From Physics to Artificial Intelligence: The Journey of Hinton and Hopfield
The journey of John J. Hopfield and Geoffrey E. Hinton from traditional Physics to AI is a story of curiosity and interdisciplinary exploration. John Hopfield started his career in Biophysics, fascinated by the principles of energy minimization in physical systems. He became interested in how neurons could interact collectively, like particles in a physical system, leading him to develop the Hopfield network. This work bridged his background in Physics with questions about natural intelligence observed in biological organisms, laying the foundation for modern neural networks.
Geoffrey Hinton, similarly, began his career influenced by the principles of Physics, particularly Statistical Mechanics. His curiosity about how the brain functions led him to explore neural networks, applying his knowledge of energy states and probability to create models that could learn from data. Hinton’s development of the Boltzmann machine was directly inspired by his understanding of physical systems and how they find equilibrium. This unique blend of Physics, Biology, and Computation was crucial in creating the deep learning revolution we see today.
Neural Networks, Physics, and the Nobel Prize: The Foundations of AI
Neural networks are mathematical models designed to mimic human brain in terms of its structure and functionality. They consist of multiple interconnected layers of nodes, like neurons, which collaborate to identify patterns in data and make informed decisions. This remarkable capability of neural networks to model and solve complex problems lead to their recognition by the Nobel Prize Committee. The awarding of the Nobel Prize in Physics to Hopfield and Hinton has sparked discussion, particularly about why work in AI, a field often associated with Computer Science, was honored in the domain of Physics. The answer lies in the deep connection between neural networks and fundamental Physics principles.
John Hopfield’s work drew heavily from Statistical Mechanics, a branch of Physics that deals with the behavior of large numbers of interacting components. In Hopfield’s model, neurons interact similarly to how particles behave in a physical system, minimizing energy to find a stable state. Geoffrey Hinton expanded on this by developing the Boltzmann machine, which was inspired by Statistical Mechanics principles developed by Ludwig Boltzmann. These networks use probabilistic nodes to explore different energy states, like atoms in a gas finding equilibrium.
This Physics-inspired approach to understanding complex systems played a crucial role in the Nobel committee’s decision. Neural network models, as developed by Hopfield and Hinton, offered a new way to understand emergent behavior—how complex patterns and intelligence emerge from simple components interacting together. This concept aligns closely with the core goals of Physics, which seeks to understand how the universe operates at both fundamental and emergent levels.
Their work laid the groundwork for modern AI, including deep learning technologies that have since led to remarkable breakthroughs. Deep learning has fueled innovations such as Generative Adversarial Networks (GANs), which allow machines to create new, realistic data by training two neural networks against each other. GANs are based on the fundamental principles of energy optimization and competition, echoing the minimization concepts found in Hopfield’s early work. Another significant leap is in Large Language Models (LLMs), such as GPT, which use deep neural networks to understand and generate human-like text. The foundational ideas of backpropagation, popularized by Hinton, are crucial to training these large models with vast amounts of data, making them capable of learning complex language patterns.
In addition, the development of AlphaFold, an AI system capable of predicting protein structures, is another milestone directly influenced by Hinton and Hopfield’s contributions. AlphaFold uses deep learning to solve the complex problem of protein folding, a challenge that involves understanding how amino acids interact—a problem deeply connected to the physical principles of energy minimization and equilibrium. Their pioneering contributions laid the groundwork for the development of AlphaFold2, which led to David Baker, Demis Hassabis, and John Jumper receiving the Nobel Prize in Chemistry in 2024 for their breakthroughs in predicting protein structures. The transformative impact of AlphaFold2 on Biology and Chemistry highlights how foundational AI research can lead to breakthroughs across scientific disciplines, including the prediction of all known protein structures and significant contributions to drug discovery and vaccine development.
The move toward Explainable AI (XAI) and Trustworthy AI also owes to these foundational concepts. While neural networks are often criticized for being “black boxes,” the understanding of internal mechanisms of a model—how it makes decisions—relates to the Statistical Mechanics principles that Hinton and Hopfield used to model learning processes. Their early work emphasized the importance of transparency in understanding how complex systems reach stable states, which is now a critical goal in making AI more interpretable, dependable, and trustworthy. Trustworthy AI ensures that these technologies are developed with fairness, accountability, and transparency, making them safer and beneficial for society.
Why Neural Networks Over Other AI Methods?
While the Physics connection helps explain why neural networks were recognized, it also raises the question: why were neural networks chosen over other innovative machine learning approaches, such as Support Vector Machines (SVMs)? Vladimir Vapnik, who co-developed the SVM model, made significant contributions to Statistical Learning Theory, and SVMs have been widely used for classification tasks and data analysis. Unlike neural networks, SVMs are built on a solid theoretical foundation that offers more interpretability, making them particularly useful in areas where understanding the decision-making process is crucial, such as medical diagnostics.
Despite their strengths, SVMs did not have the same transformative impact across diverse fields as neural networks did. Neural networks, deep learning models in particular, demonstrated an unmatched ability to solve complex problems involving massive amounts of unstructured data, such as images, speech, and text. Neural networks’ capacity to learn complex features automatically from raw data, bypassing the requirement of manual extraction of features, represented a breakthrough that enabled them to surpass other approaches, such as SVMs, across different tasks. The deep connection to Physics and their widespread impact led to neural networks being chosen for the Nobel Prize.
Economic and Social Impact
AI is already driving economic growth, and the recognition of Hopfield and Hinton’s work highlights its potential to improve productivity in various areas. AI is transforming industries like finance, healthcare, and manufacturing, creating new business opportunities, and contributing significantly to economic growth. By automating routine tasks and optimizing complex processes, AI helps companies save time and money, which drives innovation. Graphics processing units (GPUs) to speed up AI training, a technique demonstrated by Hinton, also revolutionized AI development, and helped companies like NVIDIA become tech giants.
AlphaGo, another landmark AI development, has also made a significant social impact. Developed by DeepMind, AlphaGo was trained to excel at the ancient Chinese game of Go, which is more complex than chess. Its victory over Go world champion Lee Sedol was a decade ahead of expectations, and it inspired innovative approaches in AI, showing how these systems could solve incredibly complex problems through reinforcement learning. AlphaGo’s success inspired further developments in AI, such as AlphaGo Zero and AlphaZero, which have demonstrated superhuman abilities by learning from scratch without human input. This technique may not only revolutionize competitive games like Chess and Go but could also impact games like Bridge, as seen recently when AI outperformed human bridge champions by integrating deep learning and symbolic AI. The development of such AI systems in games is a stepping stone that shows their potential to address challenges in complex, uncertain environments, and other real-world societal issues.
Beyond economic benefits, AI is changing our social lives in significant ways. The technologies developed by Hopfield and Hinton, alongside advancements like AlphaGo, are helping improve healthcare through better diagnostics, aiding scientific research, and making personalized medicine possible. These advancements promise to make essential services more accessible and effective.
Challenges and Risks in AI Adoption
Although AI offers many advantages, it also comes with considerable challenges that need careful management to guarantee its responsible use. One major concern is privacy, as AI systems typically depend on vast amounts of personal data to operate efficiently. Another significant issue is bias in AI models, where training on skewed datasets can lead to discriminatory or unfair outcomes, especially in critical areas such as employment, law enforcement, and healthcare. Furthermore, there is the risk of exacerbating social inequality, as access to advanced AI technologies might be restricted to those with ample resources, thereby widening the socio-economic divide.
The development of Trustworthy AI is essential to address these challenges. Ensuring fairness, accountability, and transparency in AI systems will help build trust and make these technologies safer and more beneficial for society. Geoffrey Hinton has acknowledged that AI’s ability to be “smarter than us” could be wonderful but dangerous as well, emphasizing the need for careful oversight and regulation to mitigate risks. However, these rapid advancements also bring ethical challenges and implications for humanity. The opacity of deep learning models, often termed as ‘black box’ AI, raises concerns regarding transparency and accountability. The unpredictability in AI systems’ decision-making processes poses risks, especially when applied in critical areas such as healthcare and governance. It is crucial to focus on the ethical development of these technologies, ensuring that AI is trustworthy and aligns with societal values to avoid potential misuse or harm.
Balancing Innovation with Ethical Responsibility
The Nobel Prize for Hopfield and Hinton is both an honor and a reflection of the transformative power of their work. It celebrates decades of relentless pursuit of knowledge, driven by curiosity and a desire to understand how intelligence functions and can be replicated. The timing of this recognition could not be more fitting. With AI now being woven into the fabric of everyday life, this award highlights the pivotal moment we are in—a time where the line between technology and humanity is constantly being redefined. It underscores the importance of fundamental research in shaping technologies that have profound implications for society.
This Nobel Prize also serves as a reminder of the responsibilities that come with these innovations. True innovation is not just about building smarter machines; it is about shaping a future where those machines empower humanity and contribute positively to our lives, while we remain vigilant about the potential risks. The story of AI is one of incredible potential, thoughtful caution, and our collective responsibility to ensure these technologies are used wisely. At this pivotal moment in technological progress, the Nobel Prize awarded to Hopfield and Hinton serves as a powerful reminder to balance innovation with a strong commitment to human values—emphasizing that technology should work for the benefit of humanity.